Understanding the Concept of Data Integration
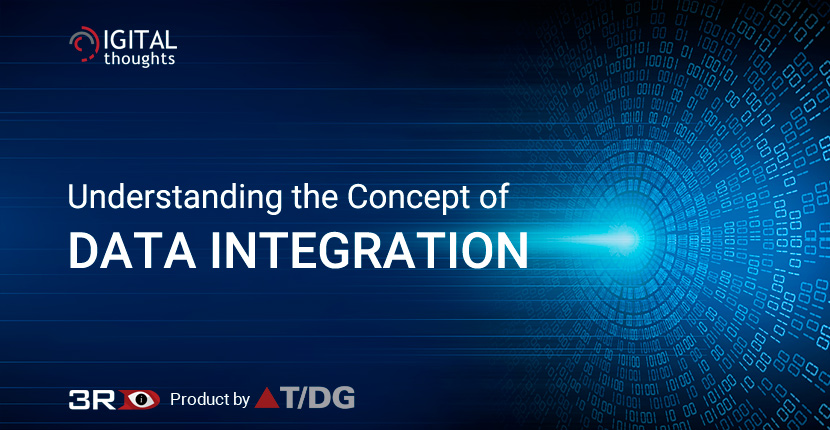
What is data integration/content integration and what is its significance in data mining? Content integration is a data preparation approach that brings together data from several sources and presents it to users in a cohesive manner. Multiple databases and flat files are examples of some of the sources of data from which data is analyzed. Building an enterprise's data warehouse is one of the most well-known examples of data integration. The benefit of a data warehouse is that it allows a corporation to do research using the data in the warehouse.
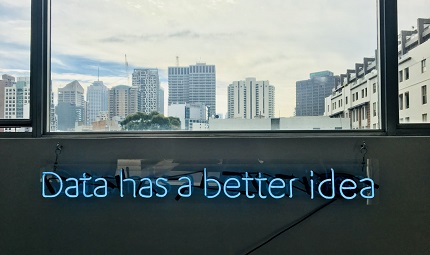
Data integration is significant because there is the need to merge data from several different enterprise structures into a single unified view, often known as a single view of the facts. This is the reason enterprises today need the enterprise search platform with data integration capabilities. This unified picture is frequently maintained in an information warehouse, which is a pertinent facts repository. Data integration is the process of combining data from many sources in order to assist data managers in analyzing it and making better business decisions. It’s all about a system that must locate, retrieve, clean, and deliver the records. Today you have the new age enterprise search platforms powered by advanced data integration capabilities.
Approaches to Data Integration
When we look at data integration, we find that there are 2 approaches, which are as follows:
a] Loose Coupling
In loose coupling facts most effectively stay in the actual source databases. In this method, an interface is furnished that takes query from the consumer and transforms it in a manner the supply database can understand after which sends the query without delay to the source databases to attain the result.
b] Tight Coupling
In tight coupling, facts are combined from specific resources right into an unknown bodily region thru the technique of ETL – Extraction, Transformation, and Loading.
Significance of Data Integration
Trying to acquire and make sense of data that reflects the environment in which an organization functions is one of the most difficult issues it faces. Every day, businesses collect an increasing amount of data in a variety of forms from a growing number of data sources. Employees, users, and consumers need a means to extract value from data in organizations. This means that businesses must be able to bring together relevant data from many sources in order to support reporting and business activities.
However, essential data is frequently dispersed across on-premises or on the cloud or provided by third parties applications, databases, and other data sources. Traditional master data, as well as new types of structured and unstructured data, are now maintained across various sources, rather than in a single database.
The conventional approach to data integration is physically moving data from its source system to a staging area, where it is cleansed, mapped, and transformed before being physically moved to a target system.
Challenges in Traditional Approach to Data Integration
The following are the issues faced by data integration, when it is done the traditional way, which are all resolved by an advanced enterprise search platform with data integration capabilities.
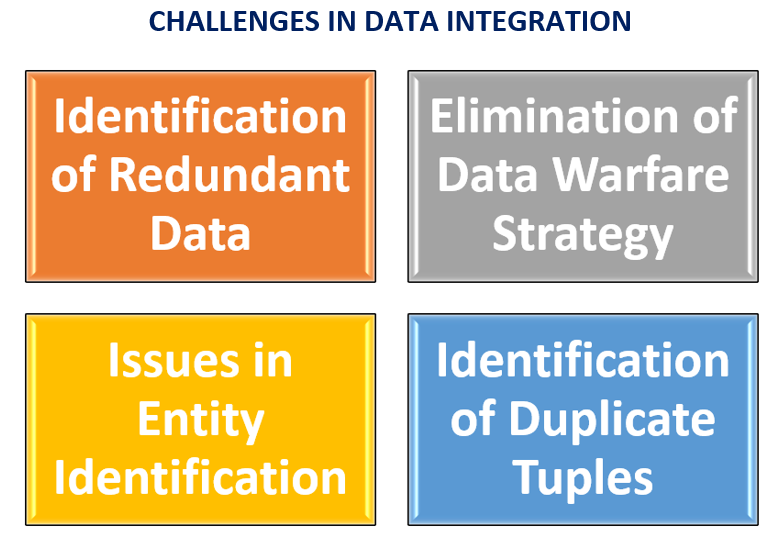
1] Identification of Redundant Data
One of the major issues encountered throughout the records integration process is redundancy. Unimportant records or data that are no longer needed are referred to as redundant data. It can also arise as a result of attributes that are derived through the use of another attribute within the data collection.
The level of redundancy is further increased by inconsistencies. The use of correlation assessment can be used to evaluate redundancy. The traits are evaluated to discover their interdependency on each difference, allowing the correlation between them to be discovered.
2] Identification of Duplicate Tuples
Data integration has also handled duplicate tuples in addition to redundancy. If the denormalized table was utilized as a deliverable for data integration, duplicate tuples may also appear in the generated information.
3] Issues in Entity Identification
Data integration is achieved by guaranteeing that the practical reliance and referential constraints of a character in the source tool match the practical dependency and referential constraints of the same character in the target machine.
A patron identity is assigned to an entity from one statistics source, whereas a purchaser wide variety is assigned to an entity from another data source. Analyzing metadata statistics will help you avoid schema integration mistakes.
4] Elimination of Data Warfare Strategy
The data warfare strategy of combining records from several sources is unhealthy. In the same way that characteristic values can vary, so can statistics units. The disparity may be related to the fact that they are represented differently within the special data units.
The solution to all the challenges mentioned above is a Data Integration platform. 3RDi Search is an example of a powerful Data Integration platform. Visit www.3rdisearch.com or drop us an email at info@3rdisearch.com and our team will get in touch with you to help you get started on your journey towards advanced enterprise search.