Understanding How the Text Analysis Engine Analyses Complex Unstructured Data
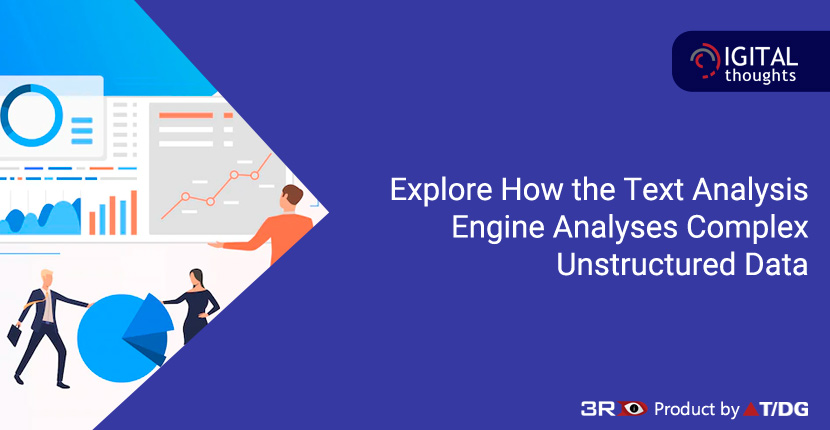
Text mining is something that every company needs today, especially at an era when companies are trying to manage massive volumes of data. Text mining tools can assist businesses in streamlining data analysis and management, as well as maximizing data's potential for corporate success. Enterprise data is a goldmine of insights that may help businesses make informed decisions that will fuel their growth and provide them a competitive advantage.
However, because most of the data is unstructured, interpreting it is difficult.
The new text analysis engine with semantic search software integration, was created with the goal of assisting businesses in extracting hidden insights from the vast volumes of unstructured company data that are constantly growing. Text analysis, also known as text mining, is the process of examining huge amounts of unstructured data in order to unearth previously undisclosed information and insights that can be used to make better decisions, among other things.
How Text Analysis Engine Works
Text mining is a complex process that combines several ideas such as statistics, machine learning, natural language processing, and more. Here we shall look at the different steps in the complex process of a text analysis engine like 3RDi Search in the analysis of complex unstructured data.
Step 1: Data Extraction
Data extraction is the initial stage in using a text analysis engine to analyze unstructured enterprise data. It entails tokenization and the identification of important terms and named entities in the data. Its goal is to turn a collection of unstructured or semi-structured data into a structured database. Data extraction is a method of searching for predetermined sequences within data using pattern matching technologies.
Step 2: Categorization
Processing, indexing, dimensional reduction, and classification are the steps that make up categorization. It's a text analysis engine concept that works on an input-output principle, with the system receiving inputs about the pre-defined categories into which the data in fresh documents should be classified. Categorization, a major feature of modern text analysis platforms, is the process of assigning one or more categories to unstructured data.
Step 3: Clustering
Clustering is a concept that brings together distinct clusters of data that have similar content. Clustering produces a number of documents known as clusters as a result of the process. The content of papers in one cluster is comparable to that of documents in other clusters, but the content of documents in other clusters is completely distinct.
Step 4: Visualization
Visualization is an idea that has been around for a long time. Uses visual signals like text flags to signify individual documents or document categories, as well as colors to show the density of a category, item, phrase, or other entity. Its objective is to improve the discovery of important information by using visual cues to organize enormous amounts of textual material into a visual hierarchy. It allows the user to scale or zoom in/out the document as needed without losing any info.
Step 5: Summarization
An important step in a text analysis engine is summarization, which is used to automatically construct a summary of the data that includes information that will be highly relevant to the user and is used to highlight the points that the user will find most valuable. To keep the meaning of the text in the summary, it employs semantic technology through semantic search software integration, comparable to that of a semantic search engine.
Wondering how you can leverage the power of an advanced text analysis engine? You need a powerful platform like 3RDi Search with semantic search software integration and powered by AI. Visit www.3rdisearch.com/ or drop us an email at info@3rdisearch.com and our team will get in touch with you to help you get started on your journey of adding more value and meaning to the experience of the user with every interaction with the text mining software.