Unlock Hidden Knowledge with Next Gen Enterprise Search Tools
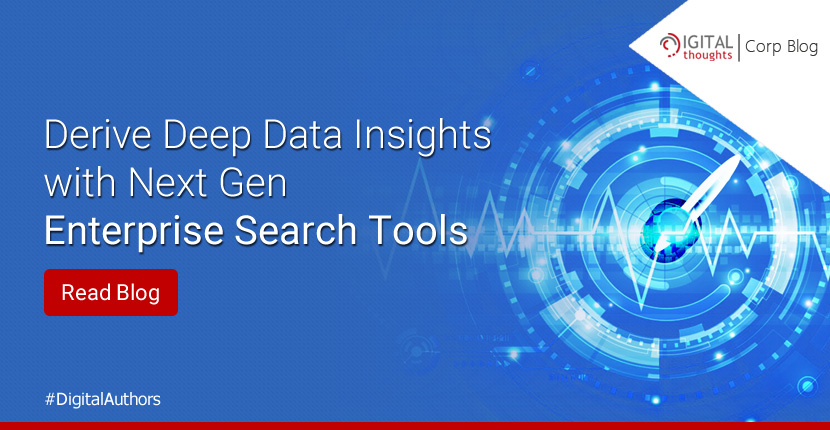
Voice commerce was a $1.8 billion retail segment in the U.S. in 2017 and $200 million in the U.K.
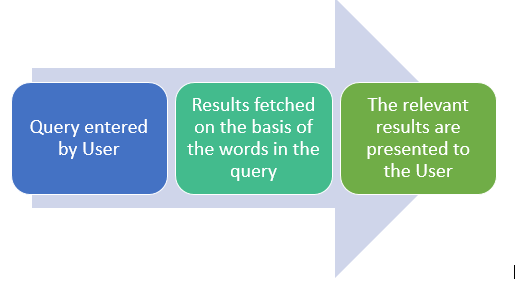
The Need for an Enterprise Search Platform…
What Makes Modern Enterprise Search Platforms so Powerful
Let’s begin with a diagram that illustrates how a modern enterprise search engine works.
Source: https://bit.ly/2CfFl53
Named Entity Recognition (NER)
Keyword Extraction
Topic Identification
Once the keyword extraction step is complete, it becomes easy to determine the topic on which the data is based. This is a huge breakthrough in the analysis of unstructured data as it allows the user to understand the topics covered within a large volume of data.
So, that was about how semantic search is the magical technology that makes it possible for the new age enterprise search tools to unlock the potential of data and provide enterprises with the insights they need to make important decisions to fuel business growth.
According to a study by Voicebot, voice search is rapidly emerging as the most popular way to search around the globe. What this indicates is that we have moved ahead (well, almost!) of the times when typing a few words into the search box was the conventional way to search. Today, search has an all-new definition and this extends not just to the quality of search results but also the way people interact with search platforms.
Search Process Before the Advent of Advanced Enterprise Search Tools…
Search technology started with the traditional keyword based approach wherein the user was required to enter a query (a query Is a collection of words that are used to find a result) into the search box and wait for the results to show up. The next step involved the search engine browsing through the database to find keyword matches (information that contained one or more words from the query), collaborate them and present them to the user. The entire process was quite linear and looked like the following:While the keyword-based approach works great for web content that is optimized, when it comes to enterprise search, this approach cannot bring the results we want. Why? The simple reason is that enterprises today deal with enormous volumes of data and a lion’s share of this data is unstructured. When we say unstructured data, we refer to data that is far from optimized and consists of data in various formats and collected from multiple sources. So, you have data that is as varied as images, videos, spreadsheets, documents and more. This kind of data cannot be analyzed using the traditional keyword based approach. However, this unstructured data is a goldmine of useful insights that can be instrumental in helping businesses make strategic business decisions. This is where a Next Gen enterprise search tool like 3RDi Search – a product of The Digital Group – can offer a solution.
Now, the question that arises is what makes the new age enterprise search tools so effective for analyzing massive volumes of unstructured data? The answer lies in semantic search technology that is used to integrate and analyze the various forms of data that most enterprise have to deal with today.
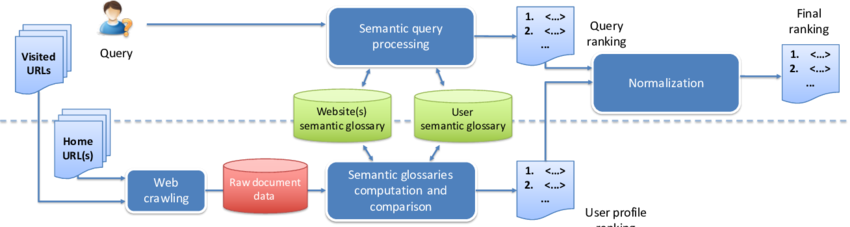
While this is a general diagram, we see that semantic query processing is the first step that the user’s query goes through. So, what is semantic search? The following are the steps involved in a semantic analysis of content:
Also known as entity extraction or entity chucking, Named Entity Extraction refers to identifying named entities hidden within unstructured data and classify them under pre-defined categories, such as names, geographical locations, departments, etc. NER is a quick approach to make sense of unstructured data that appears unintelligible.
Keyword extraction refers to the automatic identification of the terms that are the most appropriate to be used as the title of the document. An important process in text mining, it helps determine the theme or topic on which the said data is all about, which takes us to the next step.
Once the keyword extraction step is complete, it becomes easy to determine the topic on which the data is based. This is a huge breakthrough in the analysis of unstructured data as it allows the user to understand the topics covered within a large volume of data.
Semantic Classification
Semantic classification is the classification of entities in the unstructured data into pre-defined semantic classes. The semantic classes, in turn, are defined in terms of semantic features, which are nothing but conceptual components that are similar in meaning.Sentiment Analysis
Sentiment analysis is a feature that is based on Artificial Intelligence (AI) and Natural Language Processing (NLP) and involves analyzing data to determine the sentiment hidden within. In other words, a semantic search engine can analyze a piece of data to figure out if the opinions expressed by the writer is positive or negative. This is a very useful feature that allows quick analysis of data collected from social media to understand the general opinion of users about a product or a business.
For a detailed insight into how semantic search works, refer to the blog post Semantic Analysis of Text.
What’s your experience of using an enterprise search tool? Share your stories with our readers in the comment box below.